Accelerating The Journey From Edge To Cloud To Results
Presented by Inductive Automation, Cirrus Link Solutions, and Snowflake
60 min video / 52 minute read Download PDFSpeakers
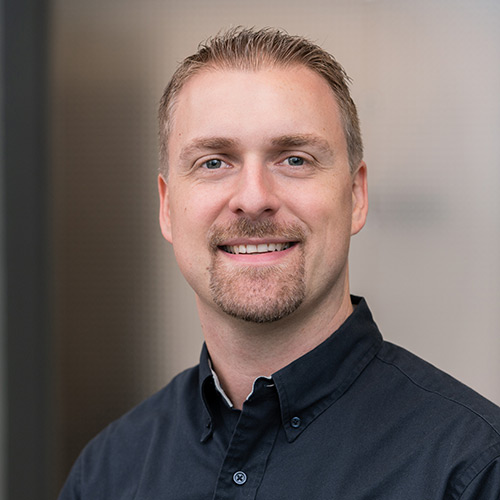
Travis Cox
Chief Technology Evangelist
Inductive Automation
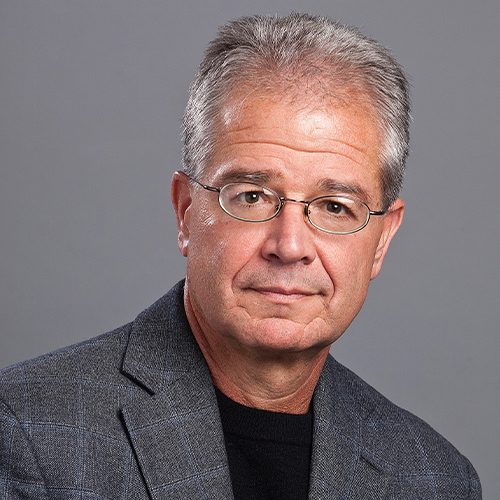
Arlen Nipper
President & CTO
Cirrus Link Solutions
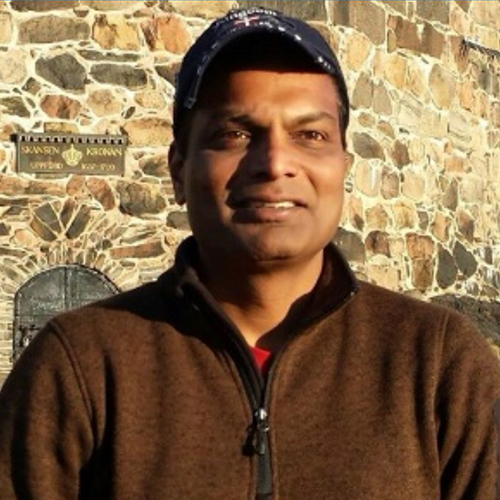
Pugal Janakiraman
Industry Field CTO - Manufacturing
Snowflake
Many industrial organizations are looking to adopt Industry 4.0 practices and face a number of obstacles. Connecting production line data, IoT, and edge device data to the cloud is very cumbersome without a plan and expensive service engagements. For most organizations, this is a very steep hill to climb.
In this webinar, find out how an integrated and proven set of technologies can make the edge-to-cloud journey much faster and easier. Industry experts will explain how to drive successful business outcomes through tools like unified namespace (UNS), digital twins, data lakes, KPI visualization frameworks for OEE and other metrics, and a lot more.
Join Inductive Automation, Cirrus Link, and Snowflake to learn about an integrated offering that turns your OT data into actionable analytics:
- Connect all your devices, collect more data, and build custom applications with Ignition by Inductive Automation
- Transfer data more efficiently with MQTT tools from Cirrus Link
- Use a single cloud platform that eliminates data silos and simplifies architectures with Snowflake
- Q&A with our experts to answer your Industry 4.0 and IIoT questions
Transcript:
0:00
Travis Cox: Hello everyone, and welcome to our webinar, "Accelerating The Journey From Edge To Cloud To Results." We are glad you've joined us here today. I'm Travis Cox; I'm the Chief Technology Evangelist for Inductive Automation, and I'm joined by two other speakers today. There's Arlen Nipper, the president and CTO of Cirrus Link Solutions, and Pugal Janakiraman, Industry Field CTO for Manufacturing at Snowflake. Arlen, Pugal, thank you so much for being here today. Can you please tell us a bit more about yourselves and your companies? Arlen, we'll start with you.
00:34
Arlen Nipper: Thanks, Travis. I'm looking forward to this webinar. Again, my name's Arlen Nipper. I'm the CTO for Cirrus Link. I've been doing automation for 45 years now. About 25 years ago, halfway through my career, I had the opportunity to work with Andy Stanford-Clark, and I was a co-inventor of MQTT. So really, Cirrus Link focuses five days a week, eight hours a day, on providing the best MQTT and Sparkplug technology for the Ignition platform.
01:09
Travis Cox: Awesome. Thank you, Arlen. How about you, Pugal?
01:12
Pugal Janakiraman: Sure. Thanks Travis. Thanks Arlen. Hello everyone, name is Pugal Janakiraman. I'm the Field CTO for Manufacturing at Snowflake. Their responsibility is to build higher-order manufacturing solutions to accelerate Digital Transformation of our customers. Again, Snowflake is a cloud company. We need an edge architecture also to make this journey happen. That's why we have closely partnered with Inductive Automation and Cirrus Link to provide this holistic solution for manufacturing customers to accelerate their Digital Transformation journey. That's what I do in Snowflake. Thanks Travis.
01:47
Travis Cox: Well, yeah, thank you both for being here today and looking forward to the conversation. So first, I wanna start here by introducing the agenda for today. We're gonna start off by talking about the benefits of MQTT and the role it plays in IoT, and the Sparkplug specification. After we'll discuss, we'll show you an extensive demo of how it all works together, and then we'll wrap up by answering any questions you have in the remaining time. If you can think of a question during the webinar, please go ahead and type it into the questions area of the GoTo Webinar control panel, and we'll get to as many of those as we can at the end. If we can't get to your question today, though, I encourage you to reach out to one of our knowledgeable account representatives, and we'll be able to answer that for you.
02:34
Travis Cox: Also, just to let you know this webinar and the webinar slides will be made available within the next few days. If you want to go over any of it again or share it with someone else who wasn't able to make it here today. Alright, to introduce, first introduce Inductive Automation. Here are a few facts about us. We make software for problem-solvers. We're focused on our software platform, Ignition. We only do software, no hardware, no services, and we focus on making the best software platform for industrial applications. We have a large footprint today, with 57% of Fortune 100 and 44% of Fortune 500 that use Ignition. So a very diversified customer base across all industries. And we have installations in over 100 countries, and we have a strong integrator program with over 4,000 integrators worldwide. We are an independently owned company, no outside investment, and our focus is on providing the best platform for our customers.
03:31
Travis Cox: And that platform is Ignition. Ignition is a universal industrial application platform to build any kind of application like HMI, SCADA, MES, IIoT, and more. It acts as a central hub for everything on the plant floor and beyond. You can use it to create any kind of industrial application. It's web managed, it's web-based, and it's web-deployed. It has unlimited licensing, and it's fully cross-platform, so allows you to deploy anywhere and allows you to scale that solution as you move forward. It offers industrial-strength security and stability. And as we'll discuss today, it unlocks you to all kinds of powerful solutions, including on-premise and the cloud, and hybrid solutions.
04:18
Travis Cox: Okay, so first, I wanna talk about some of the challenges that are there in trying to accelerate Digital Transformation. So a lot of companies are going down this path, but there are a lot of roadblocks that they're faced with. And these include things like difficulties in storing OT data in the cloud, the complexity of moving and mapping that data around, cost of transferring the data, and the lack of open standards that reduce interoperability. So when you look at the reality of Digital Transformation, the proposition looks amazing. It looks very simple: all these amazing tools, analytics, machine learning, artificial intelligence tools, all these data lakes, all these different systems that can be used. And it's like, oh, we're just gonna simply go down and get our OT data, and we're gonna make it, we're gonna bring it up there and make all... Make it possible to do more with that data.
05:10
Travis Cox: So it looks really simple, but in the reality is that we're all here in the OT space, that it's actually difficult, right? We have to look at a solution that is based on OT first; we can't cut IT down to OT. OT is gotta be where we get the data. We add that data with context, and we provide that data to other places. So what we're trying to do is, of course, make this OT world that's very complex very simple, but what's been happening with a lot of Digital Transformation projects or digital twin projects is that everything that's on-premise from an OT standpoint, whether it's the historians that are there or the live data that exists, they're thinking about, okay, that's, those systems exist. We're not gonna touch them; we're going to build other tools or use, build software programs.
06:04
Travis Cox: They're gonna tap into that data, get that data, and then manipulate it and bring it up to higher levels. And when you approach it that way, you have a lot of challenges in that you're moving data through lots of different systems. As you can see in this picture, this is a real-life kind of architecture where they, we're moving that data to the cloud, get different cloud services going into data lakes, writing lambdas or Python codes to move it into a different system to ultimately get the value of that. And when you approach it this way, the systems can become very, very brittle. And we're also not, we're not fundamentally changing the OT architecture or the OT landscape. And really, that's a big part of what we're trying to get across today is that you have to have the right foundation on the OT side, the right architecture in place, and approaching what we call the single source of truth, where you're able to define that data at the edge closest to where it exists so that it can be made available without having to map it like you're seeing here lots of different times.
07:09
Travis Cox: When you're doing lots of mapping, it does not give you real, true business outcomes. You're not getting into being able to build a UNS or any of that. You're just trying to move data around. Ultimately, we have to approach it differently, and that's the solution that we want to kind of share with you here today. The solution is leveraging Ignition and Cirrus Link to move data into a Snowflake database that allows you to store OT data with that context and that context and all that being delivered at the edge, that single source of truth to that data. The solution leverages Ignition or Ignition Edge to build, to connect to all the devices, build data models or UDTs that provide all that asset information and all that metadata and that publish that data to a cloud MQTT server using MQTT Transmission and using the Sparkplug specification.
08:03
Travis Cox: Then, from there it goes, we leverage a Cirrus Link IoT Bridge for Snowflake to move that data with that context to a Snowflake database. And where we're not having to build anything, the data is automatically stored, and those tables and structures are already automatically built out. And then from there, lastly, to build enterprise dashboards by querying that data. And we can do that in Ignition through a JDBC Driver to get that data back out. So again, if you look at this world here, quite complex when trying to do lots of mappings and try to move that data from the OT side into cloud infrastructures or into IT systems. And with this solution, it really simplifies the entire architecture. But we're also talking about leveraging tools and not coding here. So we really have two major platforms at play.
09:00
Travis Cox: We have the Ignition software platform on premise. You have the Snowflake platform for the database in the cloud, and we're leveraging open standards, MQTT, and Sparkplug to move that data into Snowflake, leveraging that IoT bridge. So you can see this architecture is drastically simplified. There's no mappings of data, and we really truly get to that single source of truth at the edge where we define that data once. And that data then is accessible everywhere else, especially getting stored to that database with that context. And then we can really truly get to business outcomes and act on that data. So what I wanna start with here is the first step in this journey, which is to connect and model the data that's on premise and how people are doing that with the Ignition platform. So Ignition is server software that acts as a hub for everything on the plant floor to kinda achieve total system integration.
10:02
Travis Cox: We can connect to any PLC. We have drivers in Ignition to lots of the major PLCs that are there, but we can also communicate over OPC UA, and with OPC, we can connect to devices that support that directly or third-party OPC servers. So we can bring in really any kind of data from the OT world into Ignition. And once that data comes into Ignition, we can then provide additional context. We can build a data model, which we'll get into in more detail. But we can also bring data from LIMs devices. Maybe it's serial devices. We can bring it in from people who are entering data into screens. We can get it from other systems through maybe web services or other maybe reading files. There's all sorts of data that you get from the OT side that you also want to be able to bring into to the business side or into a unified namespace where you could act upon that data even further.
10:54
Travis Cox: So when you look at an architecture, kind of that single source of truth architecture, a lot of companies are, of course, building their SCADA systems and are connecting to all of those devices against all different protocols that happen to be out there. And when we're bringing that data in, that is all that OT data. And by being able to publish that data to an MQTT server, even just all on-prem, you are unlocking the power of that data, and you're getting it into a format where anybody can, any application, or service can consume that information. And so this is really a decoupled architecture where the applications don't have to know about the source of the data, and the data doesn't have to know where it's going. We could ultimately publish that to an MQTT server, and there's a lot more possibilities.
11:43
Travis Cox: So you get that OT data that's now easily defined as IT data and accessible, and lots of tools can leverage it once it's in that kind of system. Now, when we look at architectures where you have remote assets or critical machines on the plant floor where you have to have local HMIs or you want to distribute your systems out, that's where Ignition Edge becomes an important part of the architecture, where you can leverage Ignition Edge out there near those PLCs, near those edge devices, connect to that data, poll it at faster rates, get more information in, and publish that data securely and by exception to that MQTT server where it can then be made available to Ignition, a centralized Ignition as a big SCADA system, or again, any other application that wants to work with it.
12:36
Travis Cox: And by doing this, we're really leveraging, we're kind of taking the brownfield world and bringing it into a modern infrastructure. And this is an important piece in terms of the... What we need to do on premise to have that single source of truth, because once we have it into this kind of infrastructure, then it makes it possible to leverage new smart sensors or new equipment that that we bring in. Especially LoRaWAN has... There's a lot of excitement around that, a lot of great sensors that are there where that data can easily be published and used, and in Ignition, where ultimately we can truly get access and define all the data we want right there, where that information needs to be, at the OT level. So these are some of the architectures that are kind of facilitating a modern architecture on premise that are allowing us to utilize that data at higher levels.
13:30
Travis Cox: Now, real quick, I wanna talk about data modeling, because this is a key part of the solution we're talking about today is that we don't just wanna push data into a database without the context, because if we do that, it's like, are we, what is the engineering units of that value? What's the accepted range? What's the desired range? What asset is that part of? We really need to know that context so that we can leverage analytics, or ML, or AI. And it really comes down to being able to... For companies to be able to define what they want their data to look like and build these models that are standardized across all of their locations. So as an example here, the bottom right, we're showing an energy data model. So it's, so we build a UDT that represents the energy of a particular load, or we can see all the different process values, and of course with those values, they have metadata like the engineering units, like the range, and so on.
14:24
Travis Cox: And what we're doing is organizing these elements of data and standardized on how they relate to each other and in terms of that asset. And a lot of times, mimicking real-world objects. But it's all about providing context to make it easy to understand. Because if we define at the edge, that's a single source of truth. If that's published up with context, then nobody has to ask the questions as to what does that data represent or mean. It is automatically understood. So data modeling is a key part, and it's been a part of Ignition for a long time, but Arlen will talk more about Sparkplug. In terms of Sparkplug has the notion of data modeling built into the specification. So it's a easy translation to build UDT and publish that up through Sparkplug, where then we can store it and leverage that context.
15:15
Travis Cox: So again, once we build that, we want to get that data everywhere. And this is really coming down to the unified namespace concept. And a lot of companies are trying to figure out how do we build, how do we get our data into a centralized repository where there's a standard way to organize and name all that data, provide that context, that structure, and have one communication interface where everybody can look at and understand, and get access to that data. And so there are lots of... MQTT has made it possible to get real-time data into a broker that many people can consume from, but a true UNS is more than just real-time data. It's about storing IT information, it's about storing OT information, it's about storing historical data. All this information we want to be able to get out, to be able to leverage and use. And that's the exciting part about the solution is we're getting, we're able to actually effectively have a UNS that customers can work with. So what I wanna do at this point is I want to kind of introduce the concepts and what we're doing there on premise with Ignition, but I want to hand it over to Pugal to talk about the Snowflake platform and what they're doing to kinda help facilitate the UNS. And so with that, Pugal, I'll hand it over to you.
16:37
Pugal Janakiraman: Yep, thanks, Travis. So before I go into what we have done for manufacturing from Snowflake's side, I'll do a quick introduction about Snowflake data platform. What does it do? Obviously, Snowflake data platform is... We are positioning that as a unified namespace for bringing together three types of data sets. There is IT data, there is OT data, there could be also third-party data, which could be traffic, weather information, depending on use cases you're trying to solve. For example, supply chain, inbound logistics, warehouse management. This data could possibly be important. But before I go there, what is Snowflake? Snowflake is a cloud-vendor-agnostic data platform. This is one of the most performant database ever invented natively on cloud. Again, I'll get into the performance numbers in a bit, but it is vendor-agnostic. A customer could be using cloud from cloud infrastructure from AWS or Azure, or GCP, or it could be mixing up any of these cloud vendors.
17:39
Pugal Janakiraman: We can manage data residing in these cloud infrastructures seamlessly for analytics without data movement, something we do really well. And you don't have to learn hundreds of services from these cloud vendors to build your own applications or leverage a cloud platform. So as far as Snowflake is concerned, it's one single platform, your interface mechanisms to go build anything in Snowflake; it's all-around SQL-based API. That's why OT world allows Snowflake because it is SQL pretty much, and there is no silos of data. You can securely collaborate on this data, and again, without data movement, for example, you could be storing some set of data in Azure. Some could be in AWS cloud, but you don't have to copy the data over from one vendor to another cloud vendor, pay for storage more than once. We leave the data wherever it is and run analytics on this and drive collaboration as well seamlessly between two different enterprises.
18:38
Pugal Janakiraman: In addition to that, we also support various types of AI and ML modeling capability. In addition to that, obviously all LLM capabilities are supported. In fact, literally a day before, we announced our own LLM called as, Arctic, Snowflake Arctic, with much higher performance, lower cost, and all those fun things, just announced a day back. But the key differentiator for us is you don't ship the data around for analytics because that leads to governance issues, security issues, and additional cost. And over a period of time leading to hundreds of repositories of data being stored around the enterprise.
19:15
Pugal Janakiraman: We move the analytics layer next to the storage and run the compute next to the data, and take the analytics and provide it to the user. So you are not sending data around. That's another major differentiator for Snowflake. And again, this is the reason we are seeing so much success in manufacturing for doing analytics around IT and OT. So having said that, to go a little bit deeper into our capability, I talked about multi-cloud nature of some customers. In fact, most of the large customers we work with have more than one cloud platform. It's pretty common for large automotive or oil companies today, if I go into it. Their product development automization could be with one cloud vendor, manufacturing and supply chain could be different cloud vendor. Their aftermarket automization could be in a completely different cloud vendor, or it could be based on regions over acquisitions, which has happened.
20:06
Pugal Janakiraman: But switching that over to one single cloud vendor is not a trivial activity for most of them, and they want to remain that way. And that's where Snowflake comes in. You can have your own cloud infrastructure in different regions in the same region for different process areas and leave the data within that environment. We allow seamless analytics of this data without data movement. That's one of the biggest differentiators we bring to the table. And even if it is a single cloud vendor, data sharing between two regions is highly complicated when, even if you use the same cloud vendor, hundreds of services involved. In the case of Snowflake, it's one single service. It is SQL API with which you are going to share data. In addition to that performance, I touched upon it. So, as I said, this is one of the most performant database ever invented today.
20:53
Pugal Janakiraman: So just to give an example, this is a data information. This happened last year, in April. For example, on a daily basis, there are 2.9 billion queries was done and in a single customer's data table, there are 50 trillion rows, and the amount of queries we do in a one-minute interval is around 160,000 queries. And just for five customers, the amount of data sitting within their customer's database within Snowflake is 177 petabytes. This is the kind of big data we handle. So bringing this large volume of OT data and IT data together to drive convergence and analytics is not an issue in Snowflake. In addition to that, the globe on the right it's not just animation, which we came up, this is literally taken out of our monitoring applications in Snowflake. Every dot represents an organization sharing data with some other organization for whatever reason, supply chain reasons.
21:49
Pugal Janakiraman: It could be traffic data, weather data being sold in our marketplace. And again, talking about marketplace, there are thousands of data vendors who are monetizing their data products in our marketplace and selling that capability to different organizations who are already in the Snowflake ecosystem. Pretty powerful ecosystem which customers are trying to leverage with data products which are agnostic of cloud data vendors underneath and sell it. You write code once on top of Snowflake infrastructure. We take care of underlying complexity, and we make sure that it is going to work for all three cloud platform. You don't have to write code three times for three different cloud vendor. We take care of it. So this is what Snowflake does. It can help you build data products and at a global scale and also monetize it. So in addition to that, now getting into manufacturing, I think Travis touched upon it. Edge-to-cloud business outcome.
22:45
Pugal Janakiraman: Every customer out there is trying to get to cloud infrastructure to leverage unlimited compute available, and a much superior AI and ML tool is to derive value out of the data. And if I categorize it in a systemic fashion, there are, at the end of the day this comes down to different types of analytics you can perform on a Snowflake data platform. It could be simple dashboarding, which falls into descriptive analytics, data visualization, whether it is OEE, cycle time, throughput, yield, common solution accelerators are required by customers. We have built those accelerators on Snowflake, and we've provided free of cost. We don't charge anything beyond the underlying compute cost we charge for customers when they use the platform. These are all the accelerators we built free of cost. In addition to that, there are diagnostic analytics capability customers are expecting, which is root cause analysis.
23:37
Pugal Janakiraman: We have LLM-powered chatbots, with which you can seamlessly navigate your data. We generate even the SQL code. The system generate the SQL code for you, given what kind of data you are expecting using LLM interface. And then after that, you can visualize the data as well using LLM capabilities. We are providing vision-based quality control solutions, and in addition to that, there are standard predictive analytics and prescriptive analytics capability customers are expecting, like predictive maintenance, predictive quality, use cases like that, energy optimization. These are all the common business outcomes customers are expecting out of Snowflake, and we have tools for doing that. Having said that, how did we do what we have done around IT/OT convergence, and what is our differentiator? To go into that, I had to go back like literally, around 18 months, when I took the role to launch manufacturing cloud.
24:27
Pugal Janakiraman: Literally, it happened a year back in Hannover Messe in April of 2023. So I realized when I took this role, we already had pretty good IT data integration capability, and that has been our claim to fame. In less than 10 years, we grew from a startup company into a $2 billion company because we provided this amazing cloud data platform infrastructure for analyzing IT data and also managing third-party data in our marketplace, where there are multiple vendors selling various data products today. So the biggest introduction which happened is last year was around OT data management. So the approach we took for solving the problem, when I say we took it, is jointly done between Snowflake, Cirrus Link, and Inductive Automation together to solve this problem. Is the first approach we took is this has to be an OT-first mindset. We cannot have a mindset where we expect the manufacturing world is going to change because, as a cloud vendor, we have come up with a cool concept that's not going to fly.
25:33
Pugal Janakiraman: We have to understand that there is 30 or 40 years of legacy in manufacturing world, multiple machine vendors, 30 to 40 different PLC vendors. There are 300+ protocols with which machines talk to each other. We have to recognize this fact, and added to that, there cannot be any coding involved to move the data from edge to the cloud with the context, because the minute you introduce coding at the edge for bringing onboarding assets, this becomes an unscalable problem. There are millions of assets, even for a single large customer writing a code to onboard an asset; it's not scalable for customers and it is too expensive. And obviously, talking about expense, we have to keep the cost low, as low as possible to democratize this OT data sending to the cloud. That's pretty much was the goal we had more than a year back, and we accomplished it around a year back, literally a year back when we launched this capability jointly with Inductive Automation and Cirrus Link.
26:28
Pugal Janakiraman: And pretty much today we have this OT data ingestion capability, which Arlen is going to demonstrate, which is completely OT-centric. As I said, it's we took an OT-first mindset, and in addition to that, this is an edge-driven, where the manufacturing intelligence and expertise resides at the manufacturing facility. Only people there know what kind of data has to be published to the cloud and what kind of analytics is going to happen at the plant floor versus what is going to happen at the edge. This happens out of edge and it has to be published by exception because OT data, as we know, the volume of data is really high. We cannot pull the network down or increase the cloud cost by sending every possible OT data to the cloud. We do publish by exception. That's what MQTT does. And added to that, we want this to be standards-based because the advantage is this brings into play every time a new device comes on board in future and which is compliant to the same standard.
27:27
Pugal Janakiraman: It is seamless to ingest this data and onboard this digital twin into cloud. That's another reason why we did this, which again, Sparkplug B is the standard we went with. As I mentioned earlier, this data democratization has to happen at scale. It cannot be coding in while you're at the edge, and it has to be at the lowest possible cost. Okay? And we achieved all of it because we use Snowpipe streaming off Snowflake, which is fraction of cost compared to any other mechanism with which you're going to send data to the cloud. Travis already talked about you have to use hundreds of services or multiple services to move this edge data to the cloud. I come across this architecture day in and day out every day because of past infrastructure decision that's been taken at the customer side. Today, with this approach, we can simplify this drastically and lower your cost and you don't have to administer multiple services; and you preserve the contextualization. And the data transfer to the cloud happens at the highest possible fidelity. Any possible data type at the edge can be modeled with the Snowflake platform today, and the data transmission happens through Cirrus Link. So that's pretty much what we have accomplished. With that, I'm going to hand it over to Arlen to demonstrate how this is all going to come together.
28:44
Arlen Nipper: Alright, thank you, Pugal. Appreciate that. So again, very quickly, who is Cirrus Link? Cirrus Link was founded in 2012 for the explicit reason to provide MQTT-centric software for industrial automation. As I mentioned, I was the co-inventor of MQTT with Andy about 25 years ago. This is the 25th anniversary of MQTT, and it was kind of frustrating that we invented MQTT for OT and then IT kind of came in and hijacked it, and we were very interested in getting it back into the arena that it was originally invented for. Now, when we started Cirrus Link, we knew that we weren't going to be able to create an entire industrial application platform. So very fortunate for Cirrus Link, we became the only strategic partner with Inductive Automation. So really, what we do on a day-to-day basis is we develop modules that run on the Ignition platform.
29:47
Arlen Nipper: Our first module that we developed in 2015 that we demonstrated at ICC was the MQTT Engine, and subsequently, we've developed an entire line of modules that run on the Ignition platform. Now we do have some standalone products as well. One is the Sparkplug-aware Chariot MQTT Broker, and the other we're gonna talk about today is the IoT Bridge for Snowflake. We worked with the Polaris team at Snowflake, and we're able to create a really optimized way to get data from Ignition through MQTT Sparkplug into the Snowflake data cloud platform. So Travis went through all the great things you can do with Ignition; it's a great HMI. You can do reports, it alarms, dashboarding all over the place. But the way that we looked at it is that we needed a tool for enterprise data connectivity. On the left side, as Travis went through before is we've got all of that connectivity to the machines in the factory.
30:51
Arlen Nipper: We're able to get all those raw register values, and we all know, we have all been working with register, PLC registers for the last 45 years. So I've got Modbus Register 40,011, and it's got a value of 17. Is that 17 gallons? Is it 17 degrees? So a human being has to sit down, and for every one of those registers, and a lot of times that's thousands and thousands of registers, we've gotta sit down and edit it to give it context, to give engineering high, engineering low, give it a name. Where was it connected in all my whole infrastructure? Now Ignition has a tool that lets us to do that. So with Ignition and the UDT Editor, we can create a data model, and then we can instantiate that, creating a digital twin. Now I know that everybody out there just cringed when I said digital twin because all the context that we have out there with what really is a digital twin.
31:57
Arlen Nipper: But what you're gonna see uniquely when I do this demo today is we're gonna create digital twins the way that we wanna use digital twins in our enterprise. We're not gonna take a digital twin that AWS invented five years ago that only gives us four pieces of information or Azure or Google Cloud Platform or somebody else because the minute we try to create a digital twin across for everybody, then nobody's gonna use it. We've got to be able to create digital twins the way that our business uses those. And, of course, we need to keep track of all the real-time data changes. Now as Travis and Pugal both mentioned, we are gonna leverage MQTT for all the reasons, security, report by exception. And then, on top of that, we're gonna utilize Sparkplug. And very quickly, Sparkplug is a specification that simply says if you're gonna use MQTT in an industrial application, this is probably a good way to use MQTT.
33:05
Arlen Nipper: So very quickly, it does four important things with an OT-centric topic namespace: it gives us plug-and-play autodiscovery. So what you're going to see is I'm gonna start with a Snowflake cloud platform that knows nothing about a smart factory, and by using the plug-and-play autodiscovery, Snowflake is going to learn about that factory in a matter of seconds. The second thing that Sparkplug lets us do is, most importantly, it lets us publish a model and an asset definition from the edge, establishing that single source of truth. So now I've got a single source of truth at the edge that's going up to the enterprise. If anything changes, we're gonna republish that data so that we always know that what the enterprise view of this system is, is always accurate. The third thing that Sparkplug does it provides us a process variable object.
34:07
Arlen Nipper: So here we've got the unified namespace coming down to the model, coming down to the process variable, so that in Snowflake, when I go look at that process variable, I immediately know where it came from. I know what machine it is attached to, and I can tell you the name, the value, the timestamp in milliseconds, data type, engineering high, engineering low, deadband, deadband percentages, scaling mode, and any other property you want to decorate that measurement with when it shows up in Snowflake. And then lastly, Sparkplug defines proper MQTT state management. With that, that means we can do report by exception, but that also means that if we should lose the network at the factory or at the facility, we can take that process variable with its timestamp and put it into a store-and-forward queue. And when our network comes back up, we can backfill that information into the Snowflake data cloud platform.
35:09
Arlen Nipper: So our network can be going up and down at all our facilities, but ultimately we're gonna be able to backfill that data into Snowflake and not having any holes in our time-series data. So really, the way that we look at it is Ignition is a platform that gives us the connectivity we need at the factory, at the remote facility, at your oil well to bring that raw data in, take that raw data, and start creating models, being able to instantiate those, creating our assets or our digital twin if you will. And that has all of our contextual data. Now, once that's in place in Ignition, the MQTT Transmission Module can look inside of Ignition, take that model and convert it to Sparkplug, and publish it to any available 3.1.1-compliant MQTT server in our infrastructure. So the resulting architecture that we're going to demonstrate here is that we're gonna take Ignition, and in there we're gonna build our data models, our digital twins, keeping track of all of our real-time data changes.
36:19
Arlen Nipper: Once we have that single source of truth, we're going to see how easy it is to then publish that to an available MQTT server through IoT Bridge and insert that, being able to do sub-millisecond insert through the Snowpipe streaming API into Snowflake. Now once I'm done with taking all this factory data into Snowflake, then Travis is gonna show you a demo of taking JDBC into Ignition Cloud Edition and being able to access that information. So right now we're gonna jump into that demo, and the first thing I'm gonna do as we go into our topology here is we're gonna go into the Ignition platform and look at building out our information. So here's my Ignition dashboard. A lot of you may already be familiar with this and using the unified namespace, you can see here. I've got a tag provider called Smart Factory, and in the Smart Factory, I've got Smart Factory 1, and then I've created a Line 1 with machines on that, Line 2. We can go down here to Line 7.
37:31
Arlen Nipper: And the way that we used to do this, you'll notice here I've got this extruder, and we would've created a folder, and then under that, we would've had all of our process variables. Now if we take a look at how we'd been doing this for the last five or six years, everybody was saying, "Okay, Arlen, we gotta get all this up to a data lake." Okay, we can do that. So let's take all of our information here and let me see here, part count. So we'll take these first floating point process variables, and we'll drag and drop those into here and literally representing our data lake. We go boom.
38:11
Arlen Nipper: Job done. There's all of our process variables in a data lake. But without context, this data lake quickly turns into a data swamp. Because if I look at this 149 degrees, well, where did it come from? And typically that means you're gonna do, okay, well, now I go do another query from another database, and I got to figure out where it came from, and then for the contextual data, I may have to query two other databases, and it's like Humpty Dumpty fell off the wall. We threw him up into a data lake, and then we spend all of our time trying to put Humpty Dumpty back together again. So what we're going to look at is, let's delete this data swamp for now. And before we do that, let's go in and using a UDT let's take the notion of that extruder machine that we had, and let's start defining what we really mean.
39:06
Arlen Nipper: And the first thing is that we probably want some asset information. So, asset ID, asset serial number, location, and then being able to go down and define that melt temperature, and seeing that, well, I don't care really where it came from, but it's going to represent 0 to 225 somethings. Those are going to be in degrees C. So all of our contextual data is defined within this model. Now, as you can see here, I've got my bunker, my chiller, my compressor, so all of my models I've defined. So we'll go back into our tags, and instead of this extruder being a folder, we can see here that is a data type extruder, and we can drill into that and see what our asset ID is, our serial number, location, then drill into the melt temperature with all of that contextual data. Now, one of the big advantages with being using models in Ignition is that gives us an easier, a very easy way to build templates to be able to visualize your data.
40:11
Arlen Nipper: So we can look at that extruder and say, "Well, that looks like our extruder." And from a process information, maybe that feeds into a bunker, and then from there it feeds into a CO2 dryer, and then we might want some energy data. So our factory energy, we've got our nice Opto 22 KYZ meter coming in, and maybe we want to measure some three-phase energy around that motor on that extruder. But really, the point here is that on Thursday, April 25th, at 11:42, this is our single source of truth, and what we want to do is we want to get that single source of truth into Snowflake. So we're going to go to either AWS or Azure Marketplace and install IoT Bridge for Snowflake, and when we do that, it's going to create two very simple databases in Snowflake. It's going to create a node and a stage database.
41:07
Arlen Nipper: And then, from here, you can see that we've built some views in, but we don't know anything about a smart factory. We don't know anything about an extruder or a bunker or a dryer or a conveyor or a haul-off. So what we're going to do is we're going to go into our Ignition, and in our transmitters, simply define our smart factory to publish to the Snowflake MQTT server, and when we do that, we're going to enable our MQTT, and what happened there is that we just established a TLS-protected outbound-only connection to an available MQTT server, and with MQTT Sparkplug, we looked into the smart factory tag provider and published that information. IoT Bridge was sitting there very quiescently, and all of a sudden, these MQTT Sparkplug messages started to arrive, and using the Snowpipe streaming, insert that into the Snowflake database.
42:12
Arlen Nipper: So a few seconds ago, we didn't know anything about a smart factory, and now if we refresh, lo and behold, there we have the Smart Factory 1, and we have views of all of the machines. Now, before I go into that view, this is all automatically created because the data in Snowflake uses the Sparkplug schema. We can go in here, and we've prebuilt a view to go in and ask the Snowflake SQL database what models have been published, and in here, oops, sorry. Node Registry, what models have been published, so we have a Node Registry, and we can see, oh, we found out about an extruder, and a dryer, and a bunker, and all of the other machines we had in that factory. We didn't have to write a single line of code, and now that we've got views of the UDTs or that digital twin, if you will, now we can come back up and look at our extruder as of view.
43:19
Arlen Nipper: And you can see here that we didn't write a single line of code, and now we've got all of our process variables, all of our columns, or high grade, with all of the real-time data that we got from the IoT Bridge from Ignition. I do have one more slide I want to go through, and that is, very, very quickly, some of the advantages that we've realized. Number one is that we're going into a Snowflake platform. That means thousands of Snowflake engineers are already knowledgeable on how to use that day one, so literally, Snowflake engineers could take what I just showed you and show you how to get business value out of that immediately. The other thing, as Pugal said, is that with Ignition, we can create 20 different data types. We need to leverage those. All other digital twins that I know of today only support a Boolean, a float, an integer, or a string, so everybody will get a copy of this. You can read through all of the other advantages of, that we see of Snowflake over any other applications out there today, and with that, I'll hand it back over to Travis.
44:39
Travis Cox: Perfect. Thank you, Arlen, for that. I want to continue off where Arlen left, where Arlen left off there, which is got all that data, all those UDT models into Snowflake with that context. All that data is coming in there, and it's stored over time. Right? Every value that's being published is going to ultimately land in that database, and once it's there, we want to be able to do awesome things with it, and from an Ignition standpoint, we can leverage Ignition or Ignition Cloud Edition, that is an AWS or Azure, to build enterprise dashboards and be able to query that data and work with that data, and discover all of those amazing assets that are there, and we can easily do that through JDBC. So you can go and download the JDBC Driver for Snowflake, install it to Ignition.
45:26
Travis Cox: We will look at, we're working on getting that bundled with Ignition by default, but with that, once you connect, you can issue standard SQL queries anywhere from Ignition to access that data, and you get incredible throughput, speed, performance. It's easy to scale. There are also REST APIs there, too, but SQL is very simple, and if you want to get into things like going into ML or the LLM capabilities that Pugal was talking about, it's certainly possible. We can leverage, there's two services that I've seen customers use right away, which is anomaly detection that's in Snowflake, and you can actually train a model by simply issuing a SQL query to train, saying, "Here's the set of data I want to train my model on." And then you can call another SQL query to call to see if there's an anomaly that was detected off of another set of data.
46:16
Travis Cox: There's also a forecasting service that they have that allows you to forecast, and they're just very easy to work with, and we're going to be publishing a resource to the Ignition Exchange that is going to show you the... That's going to be the dashboard I'm about to show you, and it'll have a screen that works with those, that anomaly detection service, so you can see how you can have a UI from Ignition that allows you to train and use those models in there. So what I want to do now is kind of show you just that last piece, which is how do we get that dashboard into Ignition? So I'm going to, at this point, we're going to come back and go over here. So here we've got Ignition on my local machine. Again, it could be on premise could be in the cloud, doesn't really matter.
46:57
Travis Cox: What I've done is I've installed the Snowflake JDBC Driver that's in here at Ignition, and then we can go make a connection to Snowflake. I've got a couple of them. I've got the Snowflake_CL, which is going to the Snowflake system that Arlen just pushed all that data to, and so we're just making that connection. Now that it's a valid connection, you know, Ignition, we just issue SQL queries, and I've got this dashboard here that, again, will be available, and when I first started, I haven't pressed the refresh button yet. When I first started, I opened this dashboard. You can see that those were the data models that were found, and Arlen now has got a whole smart factory, all that's in there. So if I refresh this, basically, we're going to see all of those data models. So we just did a query against that view that showed the registry.
47:41
Travis Cox: Here's all the models that are there. If I look at that extruder as an example over here, I can actually see here's all the parameters of that data model. Here's all the metrics for the melt temperature. There's that range that he was showing, 0–225 degrees C, and I've got one instance of it. That's the line seven extruder here, extruder seven, that we can see what those parameters are, that asset ID, serial number, and location, and see all the information. So I've discovered now this data model that is in Snowflake, and all I'm doing is issue queries against it, and from there we can then go grab that data, and we know all that context of the data. So if I go to my history here, what we just kind of, a simple screen allows us to go and query the history.
48:26
Travis Cox: And again, there'll be another screen that shows kind of the anomaly detection, and we'll get this on the Exchange. But if I go to my Smart Factory, go to Smart Factory 1, and go to that extruder, go to line seven, go to extruder seven, whatever time period I'm looking at, here's all the tags I'm interested in. I can, for the date range, I can apply, and what we're doing is we're going to go query that data and bring it back, and so here is the information for that extruder for this time period. We just query the data, and each of these different plots, the range you see there is the range of metadata of that tag. You have the engineering units over there. You get more of that context, and of course, if we want to go further and build, you know, some ML or different models off that, you certainly could.
49:11
Travis Cox: And we have, you know, a Machine Learning Manager in Ignition you could do. There's obviously great tools in Snowflake. Once the data is there in that format, it makes things so much easier to go a lot further, so hopefully it gives you a good sense of kind of what's possible there with that. Really easy to interface that data and build these kind of dashboards, again, once you have that information in there. Alright. So with that, we appreciate you guys sticking around for the demo here today. If you're new to Ignition or you haven't tried it, you can download a free trial of it on our website, inductiveautomation.com. It's quick to download. It takes about three minutes. You can use it in trial mode for as long as you want, and you can dive right in.
49:53
Travis Cox: You can try everything that we're talking about here today. You can connect to your PLCs, build UDT models, and the Cirrus Link MQTT Transmission Module and the MQTT Distributor Module is a broker, all that you can use, and then you can go play with the IoT Bridge, and you can create even a trial Snowflake account if you want to see the whole thing in action. Very easy to set this all up. We've done lots of POCs with customers, where we've got it done in really an hour. Everything's up and running, and you've got all that data with context going in there. Plus, we have lots of videos on Inductive University that are available for you, so you can learn how to connect and build those models in Ignition, how to get that published out. There's a lot of resources that are available for you as well.
50:37
Travis Cox: And just a quick reminder before we get to the Q&A, there are five days left for anybody who wants to submit your best Ignition project to our Discover Gallery for the ICC 2024, the conference this year in September. The deadline for submissions is April 30th. There's only a few days left, so if you have a project you're proud of, I'd highly encourage you to go and submit that. It's free. Getting to the Discover Gallery lets you share your innovative work with the entire community out there, and you can simply go to our icc.inductiveautomation.com and you can fill out the form. You can also get questions and email us at that what's on, with the email address on the screen here today. And afterwards, of course, for those of you if you want to get in touch, if you're outside North America, you can talk to one of our international distributors here.
51:25
Travis Cox: And you can also contact our International Distribution Manager Yegor at Inductive Automation. You can see his email address at the bottom. But we also have a lot of our account representatives that are here to help you in California, and you can also reach out to our Australia office. The number is on the screen here as well. So lots of support that's there if you want to, you wanna see a demo or get more information about this solution. Alright, with that, so we're getting to the Q&A section. We have quite a few questions that are in here already, and so the, if you guys have any more, please put them in there. So I'm going to kind of read them off here, and so the first question is, I think it's for you, Arlen. "I'm receiving pushback from security as I stated that I wanted to run an MQTT-IFM edge device connection to Hive, and they're claiming that once the outbound connection is present, it can still be taken advantage of. Can you educate me on this?"
52:19
Arlen Nipper: Yes. Outbound, so really, the security aspect of MQTT is it is an outbound connection. You're able to apply TLS or any security that your IT department wants on that, but once that MQTT is established, it is bidirectional. Now, you can put an access control on that in your broker, so all modern MQTT brokers have access control lists where you can give it a list of clients that connect, and literally, you could have all your clients have an ACL that means that they can never publish. They can only listen to MQTT topics, but in a lot of our systems, we do need bidirectional because it is a command and control system, but you can configure your MQTT brokers. If it's HiveMQ, you can configure the ACL so that no commands can be issued from the cloud side back into the edge device, if you will, at the edge.
53:21
Travis Cox: Perfect. I think another question, possibly for you here too, Arlen, is "Can you explain further how Sparkplug provides the MQTT transmission, what the function of the IoT Bridge is," and said lastly, "Compared with OPC UA, MQTT is just lighter and less complicated?" That was with the question mark, but more just kind of explain, with the IoT Bridge, the role of that product again.
53:47
Arlen Nipper: Okay. The role of the IoT Bridge is, again, we're trying to leverage a standard of getting data into Snowflake, and so IoT Bridge does two things. One, it's an MQTT client that can connect to any MQTT broker, okay, and now it can receive messages. It knows about Sparkplug, and then it's able to properly use those, convert them to JSON, and then, using the Snowpipe streaming API, insert those into the SQL database that Travis and I were showing you in Snowflake. So very simple functionality, if you will, is that it's that mechanism that you need to go from MQTT Sparkplug natively into Snowflake SQL database.
54:36
Travis Cox: Perfect. So another question here was, "Are you proposing bringing UDT in via OPC UA and then bridging to MQTT?" I can answer that. I mean, basically, any data coming in through Ignition that is represented as a UDT in Ignition can then be published to MQTT, Sparkplug, and can take full advantage of all this. Whether we built that UDT in Ignition from a different source of data or whether that was a UDT that was discovered from OPC UA, there's data modeling in some devices, either way, that data can certainly be published. And this is where OPC UA and MQTT have a lot of complement.. They complement each other in that we can take all of these, this process data and all this information, we can model it and bring it in, and then ultimately that can be efficiently set up with context and leverage in more places.
55:27
Travis Cox: So it's very exciting when you when you look at the full combination of the solution. Okay, so there's a lot of questions in here, Arlen, about, if we have simulators, if we have the environment so they can help, you know, so people can try it out themselves. I know I said I'd share that Snowflake dashboard Ignition project that's just running the queries against the schema there, but are we going to we make those, the UDT, some of the simulators, maybe publicly accessible for the community?
56:02
Arlen Nipper: Yes, we can. In fact, you and I are doing that next month with a lot of the Snowflake engineers, and so we will take the program simulators, that smart factory entire project, and put that on an Exchange so anybody can pull that down and use it.
56:20
Travis Cox: Perfect. And there's a question for you here, Pugal, which is, if they're new to Snowflake, how can they get started? And is there any kind of trials where they can play with this, you know, to see the solution?
56:35
Pugal Janakiraman: Yeah, definitely. Thanks, Travis. There are definitely multiple ways in which we can help the customers or people who are trying to do that. There are definitely trial editions available. I think the easiest mechanism is reach out to us. Pretty much, again, my contact details are available as part of this presentation, or if you have already a sales engineer or an account engineer executive assigned to your account, you can reach out to them, or you can always reach out to me. So yeah, my first name dot last name @snowflake.com. Happy to put you in touch with the right people to get this going.
57:05
Travis Cox: Perfect. And I think this is a, it's a kind of a good overarching question that, that well for both of you, it says, "What are the key benefits of incorporating Snowflake into an architecture?" But more the second question is: "Is Snowflake classified as a unified namespace or more of a hybrid data lake?" And that's something we've been talking a lot about recently. So I'll let maybe both of you answer that question.
57:30
Pugal Janakiraman: Yeah. I think I can go first. Definitely. We are positioning Snowflake as a unified namespace. It's not just a hybrid data lake, because we are bringing information with the right context into Snowflake to bridge IT and OT. I think that's pretty much what has been the crux of this demo and presentation by Arlen as well, and how that unified namespace created at the edge, which Travis showed, and that complete asset model and the OT information is preserved in Snowflake with the right context. And regarding the benefits, I think we talked about it. You don't have to manage hundreds of services to adapt to cloud. To learn cloud, you need to know only one service.
58:08
Pugal Janakiraman: It's called a Snowflake. We take care of underlying cloud vendor, different services, and managing all of it. We, in fact, reduce your data ingestion architecture from edge to the cloud drastically. Again, that showed something showed by both Travis and Arlen, which leads to reduction in cost. And also the highest possible fidelity of data. I think Arlen talked about it. There are like 13 data types needed to model edge accurately in cloud. We support all 13. Any other mechanism, you are going to go into a suboptimal approach of maybe four data types and slamming the rest on top of it. So maybe I think I'll hand it over to Arlen if he wants to add anything.
58:47
Arlen Nipper: Well, I think we've had discussion after discussion. Walker Reynolds has done a very good job in getting this notion of organizing your data. And that's really all it is. UNS is really for each company to organize their data the way they want to use it and then to be able to go access that. A lot of people are trying to make the MQTT broker in and of itself the unified namespace. And really, brokers aren't intended to do that. They're not intended to store data. They're not intended, if you start using retain, they get very cumbersome. And it dawned on me, and it dawned on Walker, and ironically, kind of at the same time, completely separately, that Snowflake really is the ultimate UNS database.
59:37
Travis Cox: Alright. So, well, that takes us to the end here of the webinar. There are definitely more questions. I'm sorry we couldn't get to all of them here today. We will follow up with those questions, or, of course, you can contact any of us to get more information about it. So we certainly thank you for attending here today. We'll be back next month with another webinar. But until then, stay connected with us on social media, subscribe to our weekly news feed email. You can also stay up-to-date through our blog, articles, case studies, and more. There's tons of useful, helpful content for you to explore on our website. So be sure to check it out. Thank you so much for being here today. Thank you, Arlen and Pugal, for joining us, providing that expertise you have and the amazing demo. And everybody have a fantastic day.
Want to stay up-to-date with us?
Sign up for our weekly News Feed.